Using AI to Streamline Messaging
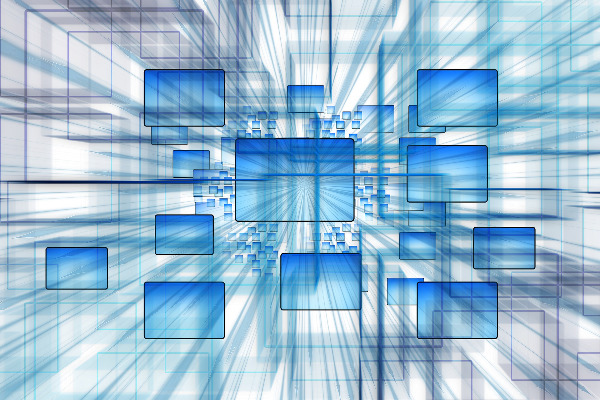
Conversational AI typically refers to leveraging bots to satisfy your customers while scaling your contact center.
At Quiq, we love bots, but we also take a broader view of Conversational AI. After all, bots are only part of digital CX.
In our view, Conversational AI also means helping your live agents work more efficiently and streamlining your operations.
In this article, we’re going to focus on how AI can (and should) be used to manage the nuances of messaging as part of a broader suite of Conversational AI.
The Need for Conversational AI
In The Nature Of Messaging, we described how messaging is a unique channel. It fluctuates between synchronous and asynchronous communication styles. It’s informal. Live agents can work on multiple messaging conversations concurrently.
All of this implies a system that can:
1. Track and prioritize the conversations assigned to a live agent.
In order to prioritize, we must understand who is expected to respond next.
2. Manage the agent’s workload.
- Keep them busy, but not too busy.
- Prioritize customers who are actively engaged.
- Move inactive customers out of the way, without losing their session.
3. Map free-flowing streams of messages into tickets in traditional CRM systems.
In order to achieve the above, you need a purpose-built system (like Quiq) that handles the fluctuating synchronicity of conversations amidst the backdrop of agent concurrency. The system is also going to need a hefty dose of AI to do the best possible job.
Let’s consider some examples to explore why.
Here’s a pretty typical inbound service conversation that was routed directly to a live agent.
The agent sent the last message, but is it the customer’s turn to respond?
No.
The agent essentially promised a follow-up. The system should still prioritize this conversation.
The traditional algorithm employed in email management systems is that the two parties should take turns, but that doesn’t work in conversational settings because messages are shorter and less formal.
We need NLP/AI here.
Here we have the opposite situation. The system shouldn’t prioritize this conversation or set any sort of SLA timer because the burden of response is on the customer.
If the customer fails to follow up within a reasonable timeframe (10 minutes?), the system should move this conversation to an inactive state to make room for customers who are more engaged.
Do you remember choose your own adventure books? In this example, you get to pick what happens next:
- Nothing. The conversation is over.
- An hour passes. The customer responds with “You too!”
- An hour passes. The customer responds with “Actually, I don’t want green after all.”
- An hour passes. The customer responds with “I have a different question for you.”
Compared to phone and email, it’s less clear when a messaging conversation is actually over.
Obviously, we don’t want to just leave the conversation open; that delays helping other customers.
So the system should automatically inactivate and/or close it.
If scenario 2 happens, what should we do?
We definitely don’t want to open another tracking ticket, and we may not even want to reopen the conversation and route it to the agent (especially if that agent isn’t online anymore). We call this the ‘long goodbye’ problem, or more generally, an unimportant response.
If scenario 3 happens, we need to reopen the conversation and it should be associated with the same ticket in the CRM and ideally routed to the same agent.
If scenario 4 happens, we should start a new conversation associated with a new ticket and route the conversation to our entry point (e.g. a bot) rather than directly routing to the agent.
In messaging apps, there isn’t a clear start and end point to a conversation—and there isn’t an equivalent of an email ‘Subject’.
It’s just a stream of messages with potentially long delays between them. So in order to solve the above problems, we need a deep understanding of the message content.
The Impact
The examples we explored above aren’t just academic. They’re impactful to your operations.
Consider the following stats taken from across our user base (your org’s exact numbers might differ):
10% of conversations will have a late, ‘unimportant’ message arrive.
- Failure to recognize these as continuations of the previous conversation causes superfluous records that impact analytics.
- Agents are unnecessarily distracted.
The traditional ‘take turns’ response algorithm is wrong 30% of the time in messaging.
- If we fail to prioritize a conversation where the customer is expecting a response, we risk missing SLAs and angering customers, while forcing agents to attempt their own prioritization.
- If we prioritize a conversation that is actually waiting on the customer, we decrease efficiency by distracting the agent and delaying service to other customers.
20% of your messaging conversations will reopen in a 72-hour period.
It’s important to recognize when an important message arrives and determine if it represents a new topic or a continuation.
Our Approach
At Quiq, our goal is to leverage AI to have a positive and immediate impact on our customers and their businesses.
We follow the latest research and pragmatically adapt and apply AI to the context of conversational business messaging.
For the majority of our AI modeling tasks, it’s not sufficient to simply consider the text of a single message in order to make a decision.
Instead, we must consider all of the recent transcripts, including the sequence of individual messages and their authors. This deep understanding of the conversation transcript enables us to achieve high accuracy on problems like the ones presented in this article.
Stay tuned as we build out more!